Explainable Road Hazard Detection via Pixel-wise Uncertainty Analysis
Dec 11, 2024
·
1 min read
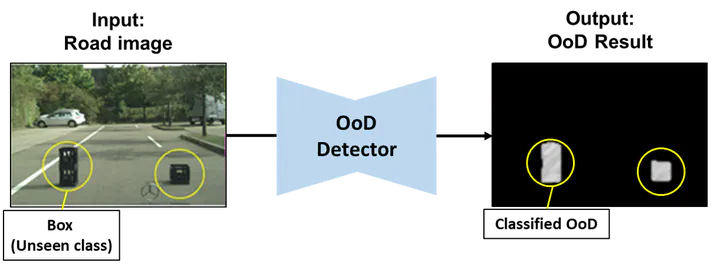
📸 Gallery
Pixel‑wise logit variance drives a segmentation network that spots debris and potholes while quantifying risk for autonomous vehicles.
Highlights
- Uncertainty‑aware segmentation improves average precision by 21.7%
- Iterative background refinement sharpens hazard edges
- Bench‑tested on 180 k Korean dash‑cam frames; 45 FPS on RTX A6000
Publications
- Road anomaly segmentation based on pixel‑wise logit variance with iterative background highlighting, ICRA 2023
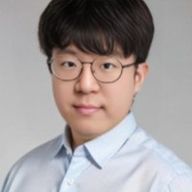
Authors
Ph.D. AI Researcher | XR Simulation | Explainable AI | Anomaly Detection
I am an AI researcher with a Ph.D. in Computer Science at KAIST, specializing in Generative AI for XR simulations and anomaly detection in safety-critical systems.
My work focuses on Explainable AI (XAI) to enhance transparency and reliability across smart infrastructure, security, and education.
By building multimodal learning approaches and advanced simulation environments, I aim to improve operational safety, immersive training, and scalable content creation.
My work focuses on Explainable AI (XAI) to enhance transparency and reliability across smart infrastructure, security, and education.
By building multimodal learning approaches and advanced simulation environments, I aim to improve operational safety, immersive training, and scalable content creation.